GLCM Cross Transformation¶
An alternative to GLCM statistics within an image, is to take statistics across images.
With similar requirements to Batched GLCM
, images participating must be of the same shape.
Combinations are between the Channel Dimension, while independent of the Batch Dimension.
Remember that the expected Input Shape is: B x H x W x C or H x W x C
from matplotlib import pyplot as plt
from matplotlib.image import imread
from mpl_toolkits.axes_grid1 import ImageGrid
Read Image¶
ar = imread("../../../data/image.jpg")[:250, :250]
plt.imshow(ar)
<matplotlib.image.AxesImage at 0x1f5774f9af0>
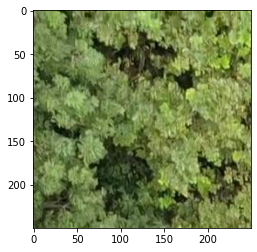
Theory of Cross GLCM¶
We find all pair combinations in the channel dimension.
Here, we see that ar
has 3 channels.
Thus the combinations are
[Channel 0, Channel 1]
[Channel 0, Channel 2]
[Channel 1, Channel 2]
Combination Order¶
To inspect the combination order, you can find them using itertools.combinations
ar.shape
(250, 250, 3)
from itertools import combinations
list(combinations(range(ar.shape[-1]), 2))
[(0, 1), (0, 2), (1, 2)]
from glcm_cupy import GLCMCross
radius = 2
gc = GLCMCross(bin_from=256, bin_to=16, radius=radius)
ar_gc = gc.run(ar)
print(ar_gc.shape)
GLCM Progress: 94%|█████████▎| 170k/182k [00:00<00:00, 330k Cells/s]
(246, 246, 3, 7)
As above:
ar_gc[:,:,0,:]
corresponds to the cross between Channel 0 and 1.ar_gc[:,:,1,:]
between Channel 0 and 2.ar_gc[:,:,2,:]
between Channel 1 and 2.
Plot Transformations¶
Note how we find the pair indices via itertools.combinations
# Plot in a grid
fig = plt.figure(figsize=(15, 20))
grid = ImageGrid(fig, 111,
nrows_ncols=(7, 3),
axes_pad=0.4)
ax_ix = 0
for f_ix, title in zip(range(ar_gc.shape[-1]),
("HOMOGENEITY", "CONTRAST", "ASM", "MEAN",
"VARIANCE", "CORRELATION", "DISSIMILARITY"), ):
for e_ch_combo, ch_combo in enumerate(combinations(range(ar.shape[-1]), 2)):
ax = grid[ax_ix]
ax.imshow(ar_gc[..., e_ch_combo, f_ix] ** (1 / 3))
ax.set_title(f"{title}, {ch_combo}")
ax_ix += 1
fig.suptitle('GLCM Cross Features (Cube Rooted for visibility)')
Text(0.5, 0.98, 'GLCM Cross Features (Cube Rooted for visibility)')
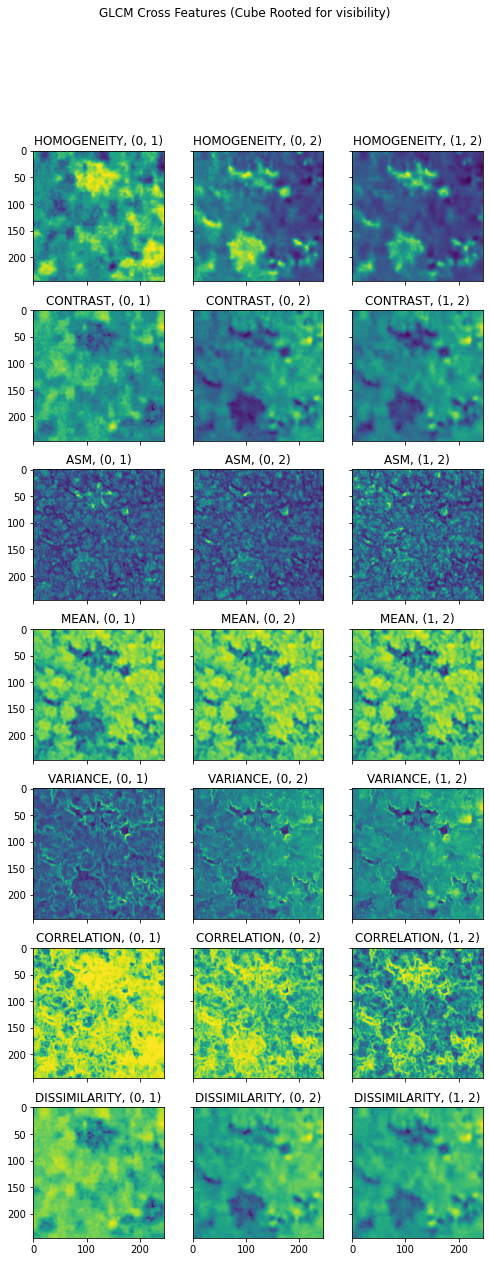
Summary¶
glcm-cupy
works with batched images, for convenience and a small speed up!